Data Science
24 Weeks
- Expert Trainer
- Affordable Fees
- Placement Opportunities
- Hands on Training
- Flexible Timings
- Industry Based Training
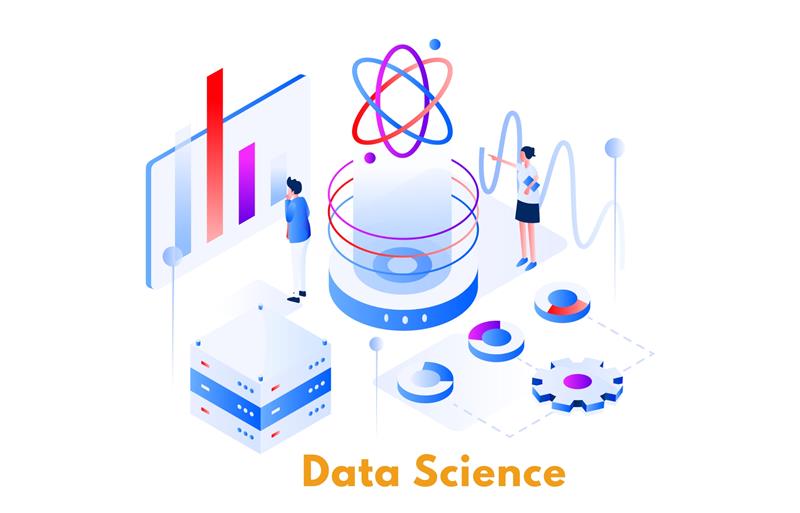
Overview
Zappcode Academy’s Data Science Course is designed for beginners and professionals who want to build a strong career in data science. The course provides comprehensive training in Python, SQL, machine learning, and data visualization using real-world projects. No prior coding experience is required to start your data science journey.
Our curriculum follows a practical, hands-on approach where students learn to analyze, interpret, and visualize data to make data-driven decisions. With tools like Tableau, Power BI, and Scikit-learn, learners gain experience in solving real business problems using data science techniques commonly used in top tech companies.
The course is taught by experienced data scientists who bring industry knowledge and mentoring to the classroom. Students receive lifetime access to learning materials, personalized career guidance, and placement support. Zappcode Academy ensures every student graduates with the confidence to apply data science skills in the real world.
Attend Free Demo
Fill the details and we will call you for further guidance
What You’ll Learn in Our Data Science Course
By the end of this course, you will:
1
Understand the fundamentals of Data Science and Machine Learning
2
Clean, analyze, and visualize large datasets using Python and Pandas
3
Work with real-time data and build predictive models
4
Use SQL to query structured data from relational databases
5
Build dashboards and reports using Tableau and Power BI
6
Implement Machine Learning algorithms using Scikit-learn
7
Understand Data Preprocessing, Feature Engineering, and Model Evaluation
8
Get hands-on experience with real-world capstone projects
Our course structure emphasizes practical application so you’ll graduate with a portfolio of real projects.
Course Framework
Module 1
Introduction to Data Science
What is Data Science?
Lifecycle of a Data Science Project
Career Path and Scope
Module 2
Python Programming Essentials
Python Basics & Control Structures
Functions and Core Syntax
Module 3
Data Handling with Python
NumPy and Pandas
Data Wrangling and Manipulation
Module 4
SQL for Data Analysis
SQL Queries, Joins, Aggregations
Subqueries and Window Functions
Module 5
Data Visualization
Matplotlib & Seaborn
Tableau Dashboards
Power BI Reports
Module 6
Statistics and Probability
Descriptive & Inferential Stats
Probability Distributions
Hypothesis Testing
Module 7
Machine Learning Fundamentals
Supervised & Unsupervised Learning
Regression, Clustering, Trees
Model Evaluation
Module 8
Capstone Project
End-to-end real-time project
Model deployment & presentation
Tools & Technologies Covered
At Zappcode Academy, we train you on the most in-demand tools used by data scientists worldwide:
Programming
Python, R (optional)
Libraries
NumPy, Pandas, Matplotlib, Seaborn, Scikit-learn
Visualization Tools
Tableau, Power BI
Databases
MySQL, SQLite
Version Control
Git & GitHub
Cloud & Deployment (Intro)
Google Colab, Streamlit
All tools are taught from scratch. No technical background? No worries – we cover it all.
Instructor Profile – Learn from Seasoned Industry Experts
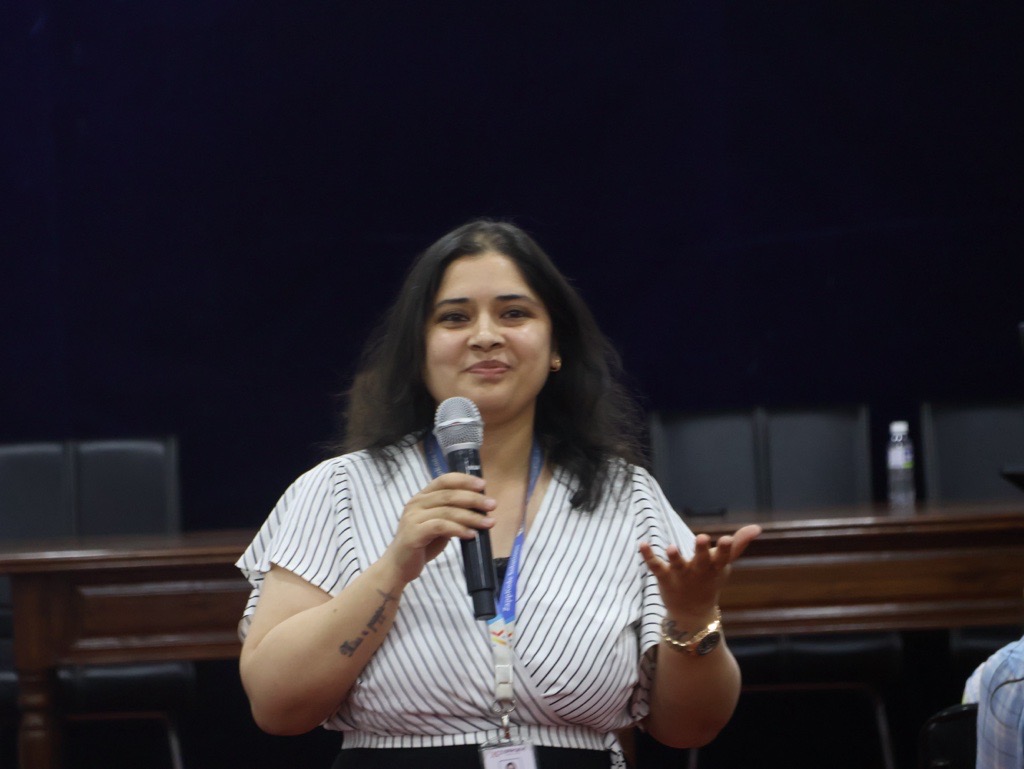
Rashmi M. Joshi
Co-Founder & Lead Technical Trainer, Zappcode Academy
Rashmi M. Joshi, co-founder of Zappcode Academy, is an experienced IT educator with 9+ years in the tech industry. She specializes in hands-on training and project-based learning, combining real-world experience with education. Rashmi has led numerous projects in ERP, web/mobile apps, SaaS, and business automation, and is known for her strong mentorship in career planning and interview preparation.
Technologies she works with:
Data Science
Python
SQL
Git & GitHub
Full Stack Development
Verified Achievement
Validate your learning with proof of achievement
Boost your chances for job opportunities and promotions
Frequently Asked Questions (FAQs)
The course duration is 6 months, including weekend and weekday batches.
No, our course is beginner-friendly. We start from the basics of Python and gradually build up to advanced topics.
Yes, you will receive a Data Science Course Completion Certificate from Zappkode Academy, plus a project/internship certificate.
Yes, we provide placement support, resume building, and mock interviews to help you crack your first data science job.
Our Data Science Course is affordably priced, with flexible installment options. Contact us to get the latest fee details.